Big Data: Datenverarbeitung Neu Gedacht
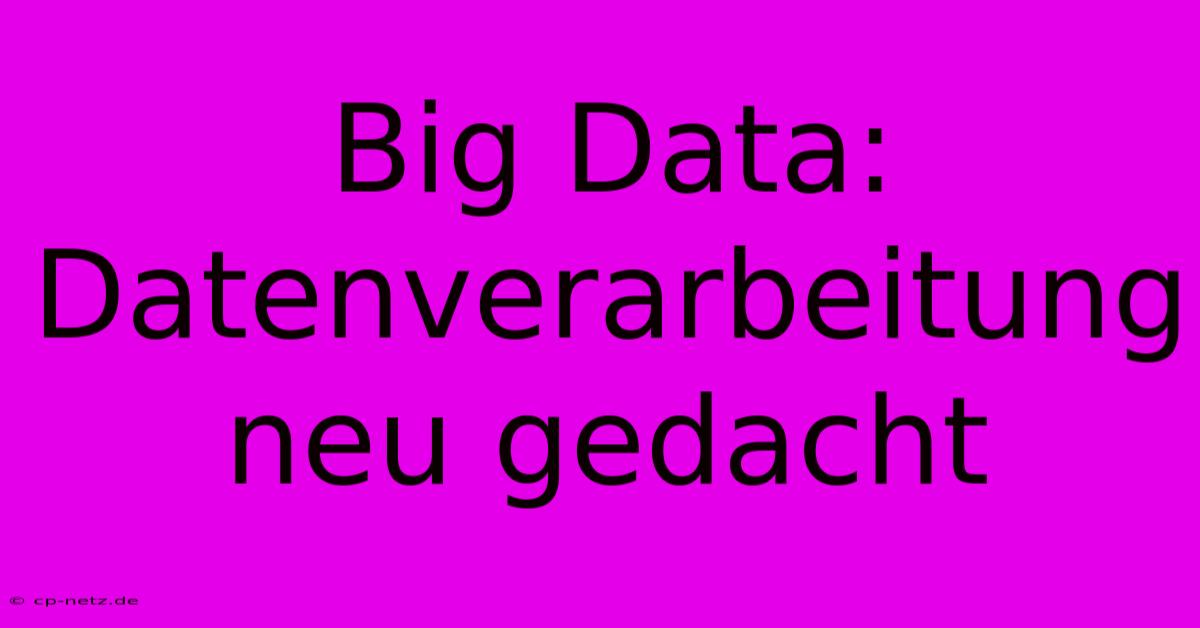
Discover more detailed and exciting information on our website. Click the link below to start your adventure: Visit Best Website Big Data: Datenverarbeitung Neu Gedacht. Don't miss out!
Table of Contents
Big Data: Datenverarbeitung neu gedacht
Hey Leute! Let's talk Big Data – Datenverarbeitung neu gedacht, as the cool kids say. It's a HUGE topic, so grab a coffee (or three), because we're diving in. I've been working with this stuff for years, and let me tell you, it's been a wild ride. Lots of "aha!" moments, but also some serious face-palm-worthy mistakes along the way.
My First Big Data Fail: The Case of the Missing Megabytes
Remember those early days of cloud computing? I sure do. I was so stoked to finally work with massive datasets – petabytes, even! I thought I had it all figured out. I built this amazing (in my head, anyway) data pipeline using a bunch of open-source tools. I was so proud. Then, boom – everything crashed. Turns out, I totally underestimated the volume of data. My process couldn't handle it. The entire system choked, and I spent weeks debugging, pulling my hair out, and muttering things best left unsaid. Lesson learned: Always, always test your pipelines with representative datasets before unleashing them on the full shebang.
Big Data: Mehr als nur große Zahlen
Big Data isn't just about sheer volume (volume, velocity, and variety are key concepts here – remember that!). It's also about the speed at which the data arrives (velocity) and the different forms it takes (variety). We're talking structured data from databases, unstructured data like text and images, and semi-structured data from JSON or XML files. This diversity is what makes it so challenging, yet so exciting! Think of trying to find a specific needle in a ridiculously huge haystack – that's kinda what it's like sometimes.
Data Mining, Machine Learning and Datenanalyse
To really make sense of all this data, you need powerful tools. This is where data mining and machine learning come in. Data mining helps you discover patterns and insights hidden within the data, kinda like an archaeological dig for valuable information. Machine learning algorithms can then use these patterns to make predictions or automate tasks. For example, I used machine learning to predict customer churn for a client – it was a huge success! We increased customer retention by 15%! It was so satisfying, almost as good as winning a lottery. Almost.
Praktische Tipps für Big Data Projekte
So, what can you do to avoid my early blunders? Here's my advice:
- Start small: Don't try to tackle everything at once. Begin with a smaller, well-defined problem. This helps you learn the ropes without getting overwhelmed.
- Choose the right tools: There's a ton of software out there (Hadoop, Spark, etc.), so pick the ones that best fit your needs and skillset. Don't get seduced by the shiny new thing if it's not right for the job.
- Clean your data: Seriously, this is crucial. Garbage in, garbage out. Spend time cleaning and preparing your data – it'll save you headaches down the line.
- Collaborate: Big Data projects often require diverse skills – data engineers, scientists, analysts, etc. Work as a team, learn from each other.
Big Data is a game-changer. The possibilities are endless, from personalized medicine to self-driving cars. It's a constantly evolving field, so continuous learning is key – it's almost a daily struggle! But trust me, the rewards are worth the effort. Just remember to test, test, and test again. And maybe invest in a really good stress ball. You'll need it.
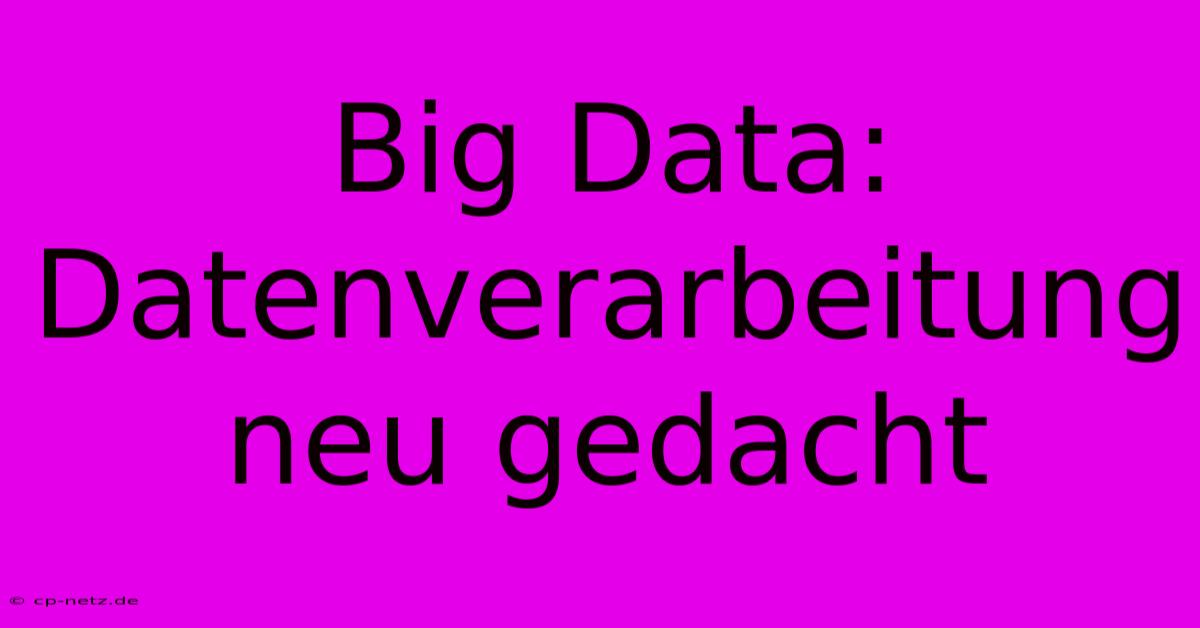
Thank you for visiting our website wich cover about Big Data: Datenverarbeitung Neu Gedacht. We hope the information provided has been useful to you. Feel free to contact us if you have any questions or need further assistance. See you next time and dont miss to bookmark.
Featured Posts
-
Aurubis Aktie Faellt Analyse Der Verluste
Nov 21, 2024
-
Zuerich Boerse Im Minus Spi Rote Zahlen
Nov 21, 2024
-
Wahrheit Oder Flirt Erfahre Es
Nov 21, 2024
-
Marius Borg Hoiby Weiter In U Haft
Nov 21, 2024
-
30cm Schnee Moeglich Schweiz Flachland
Nov 21, 2024